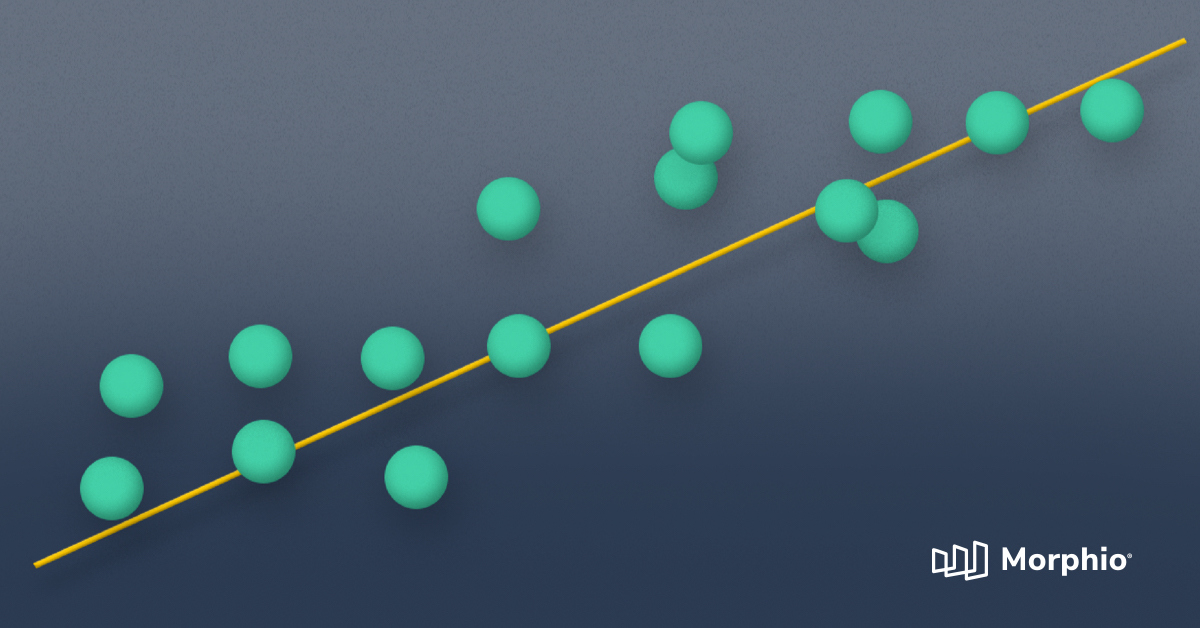
If you’ve ever approached deep learning, you’ve come across two methods to train algorithms: supervised and unsupervised.
Think of them like when you put together IKEA furniture. There are different ways you can approach it and (hopefully) it results in a completed couch or chair. Some approaches make more sense than others too.
Have the instruction manual and the right pieces? Great, follow directions. Don’t need the manual anymore? Toss it aside.
Machine learning is the same. Depending on the kind of data you have available and the end goal, how the algorithm should be trained changes.
Let’s walk through what supervised and unsupervised learning looks like and when to use them.
Start Defending Against Marketing Failures Today!
Discover why over a 1000 businesses trust Hawke AI to help protect their marketing ROI.
Request a free trialWhat is machine learning?
Let’s take a couple of steps back.
Firstly, what is machine learning?
We like to describe it as a form of artificial intelligence that turns data into knowledge and decisions. Or, a little friendly robot that gives you recommendations.
It uses our input to train models and algorithm which are capable of becoming smarter over time to make increasingly better changes or suggestions.
Machine learning also finds patterns and trends within datasets so businesses can make smarter judgments without bias or emotion.
You have a bunch of apples, bananas, and oranges. A simple example, I know. But keep with us.
That goes through the machine learning software for interpretation to train an algorithm that processes the input.
The output is patterns found within the data, neatly organized for businesses to leverage.
But, what if you wanted to control the output? What if you wanted AI to find patterns without us giving it explicit labels?
That brings me to my next thought.
What is supervised machine learning?
Supervised learning is typically done in the form of classification or regression.
The goal is to find relationships or structures within data input to effectively correct the output. It’s like holding a child’s hand (a really smart one!) You let it do some work, but you guide it in the right direction.
The classification type of supervised learning is used to predict which class a data point will be a part of and its value. Forecasting whether a customer will convert or not and how much revenue they will generate could be considered classification.
Regression, on the other hand, is used to predict quantity output. Continuous output variables are integers or floating points like what will revenue be tomorrow or the amount of cash flow available next Thursday.
The marketing security software we offer for marketers at Morphio is a great example of supervised machine learning using regression. In particular, we use it for anomaly detection.
Anomaly detection has emerged as an efficient ‘hands off’ approach to catch marketing failures such as landing pages going down, tracking breaking or ads getting paused.
Anomalies can also highlight growth opportunities, but let’s stick with fixing problems for now.
You can see that Morphio’s anomalies are presented as cards (using a combination of regression) and standard deviation. Level one is a minor anomaly while three is very unusual and therefore critical for an intelligent human to review.
We use data collected from Google Ads, Google Analytics, and our other integrations to train models with a set goal. (Hence why it’s considered supervised.)
The band with the inside dotted line is the range that data is expected to fluctuate within. The dots are real performance and hovering over one shows its value.
You can also leave a comment to start discussions with team members.
But enough about that. Let’s talk about unsupervised learning next.
What is unsupervised machine learning?
The opposite of supervised learning is unsupervised: learning the structure of our data without the use of explicit labels.
It’s very beneficial in exploratory situations because it automatically identifies patterns in datasets.
Let’s say that we wanted to segment customers to find profitable audiences.
Unsupervised methods would be excellent because an algorithm can provide initial insights to test hypotheses. Us clunky humans can’t keep up with that speed and our ideas are usually based on hunches—not good for business!
Unsupervised machine learning also uses something clustering to achieve this.
Imagine two datasets on an X and Y-axis.
Machine learning helps find patterns then groups them into clusters. Here’s a visualization:
We could collect a huge customer base and unsupervised learning would group segments (clusters) based on traits like lifetime value, retention rate, and others it discovers.
You can’t be guessing. You need accurate data.
That’s where unsupervised machine learning swoops in like Superman.
It gives marketers insights that they may have not have found otherwise thanks to the speed and power of this technology.
And the reality is that old data is fubar. You have enough of it already. Organizations can remove bias with machine learning software to make better business decisions faster.
Once unsupervised learning has labelled data into clusters, businesses can then begin interpreting it and testing hypotheses.
Supervised vs unsupervised machine learning conclusion
Figuring out how supervised vs unsupervised differs can be a real headache. Let me spare you some Asprins.
Supervised machine learning is training models with a target. You give it the data, let it train models, but at the same time control the destination and what it’s going to give back. This uses classification and regression types.
It can be used to forecast lifetime value, customer segments, churn, and other key metrics of business performance. A.K.A it separates the datasets and gives it a value based on a target.
Unsupervised machine learning, on the other hand, uses clustering and is not given a target. Rather, it uses the initial data to find underlying structures and patterns which are extracted and labelled.
It can be used in marketing or sales to take unlabeled data and uncover trends that you wouldn’t otherwise catch. These can be things like profitable customer segments, next best actions, and ad targeting.
Want to experience all of those benefits? Try Morphio today for free for 14-days. Our unique platform uses a regression-based model to find profitable opportunities and marketing failures before humans can.